Tl; dr;
- Understand motivations: Go beyond observable actions by exploring the reasons behind customer behaviors. This can reveal motivations that drive purchasing decisions, providing valuable context for data-driven strategies.
- Customer segmentation is key: Categorize customers effectively to tailor marketing and product development. Segmentation based on behavior, demographics, and psychographics enables more personalized and impactful interactions.
- Patterns lead to predictions: Detecting recurring behaviors within customer data is crucial for anticipating future trends. Use these patterns to forecast needs and preferences, guiding proactive business decisions.
- Online surveys minimize user friction: Utilize online surveys to collect customer feedback efficiently. This method reduces customer barriers to sharing experiences, leading to richer data and more accurate analysis.
- Quantitative meets qualitative: Blend numerical data analysis with qualitative insights to comprehensively understand customer behavior. This combination allows for a more nuanced approach to interpreting and responding to consumer needs.
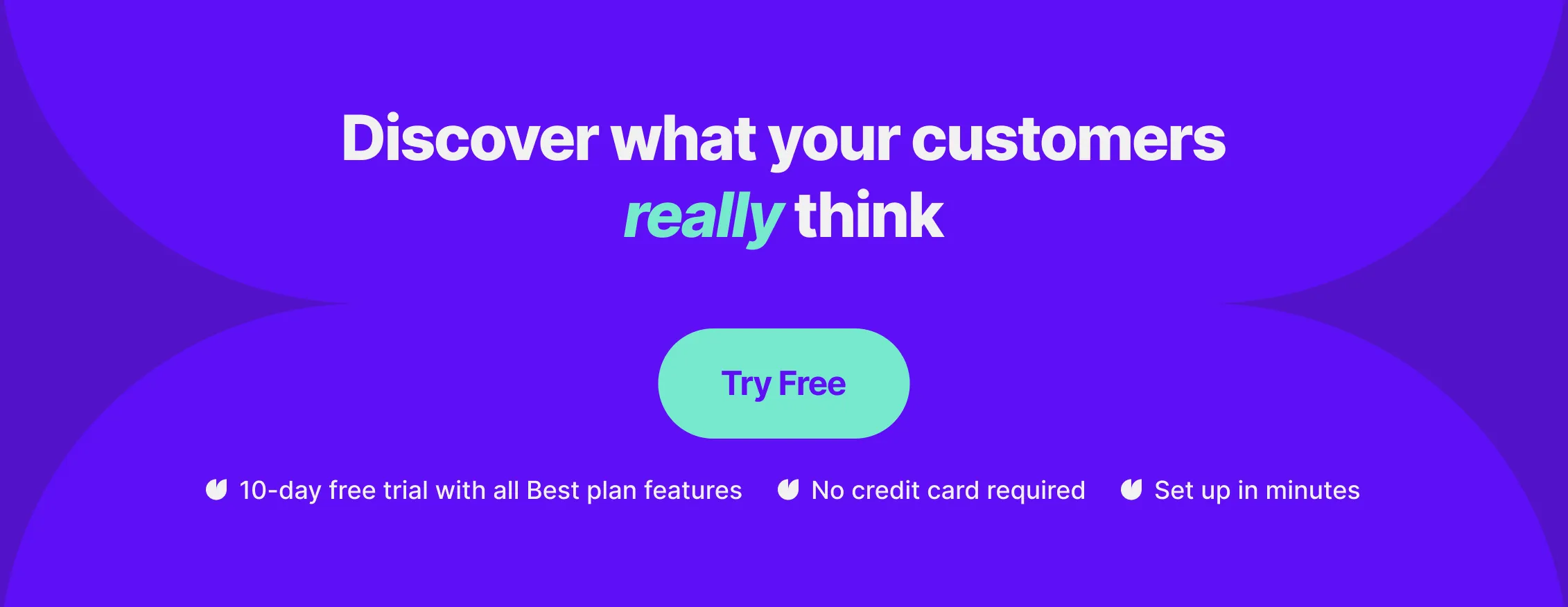
Ever felt like you needed a crystal ball to understand what your customers really want?
While we can't offer you magical powers, we can certainly equip you with the next best thing: a guide to customer behavior analysis.
We’ll go through different sources of customer data, uncovering patterns and preferences that can transform your business strategy. The aim is to anticipate customer needs, tailor your offerings, and ensure your customers keep returning for more.
Read on ⤵️
What is customer behavior analysis?
Customer behavior analysis is a way to understand how your customers interact with your business. It incorporates collecting and examining data related to purchasing habits, interactions, product usage, and customer feedback.
Key elements of the customer behavior analysis
- Data collection: Gathering qualitative and quantitative data from various customer touchpoints.
- Customer segmentation: Grouping your customers based on demographics, interests, or behaviors.
- Pattern identification: Looking for common elements within segments, such as frequent purchases or preferred content.
How to conduct a customer behavior analysis?
- Identify segments: Look at user properties like demographics and interests.
- Data examination: Assess the gathered data for behavior patterns.
Effective customer behavior analysis can improve online customer experiences by predicting trends and making better business decisions. It's a blend of observing what customers do and discerning why they do it, which involves understanding both their conscious and unconscious motives.
- Analyze: Use tools to process and understand data patterns.
- Predict: Make informed guesses on future customer behavior.
- Act: Implement changes aimed at enhancing customer satisfaction and driving revenue.
Data collection methods
Effective data collection is indispensable for customer behavior analysis. Various methods are available, each offering unique insights.
Online surveys
You can run online surveys to gather quantitative and qualitative data. Rating scales, for example, the Likert scale, will help you to collect more statistical data, while open-ended questions will provide more depth and context to the customer feedback.
💡Tip: Use tools such as Survicate, offering various distribution channels, AI surveys and feedback categorization to speed up the research process, and numerous integrations with other software from your tool stack.
Online reviews
Online reviews are a valuable source of qualitative data. They are spontaneous opinions voluntarily shared by customers and should be included in the analysis of customer behavior online.
💡Tip: AI-powered features such as Survicate’s Insights Hub can dramatically improve analyzing large amounts of such information in a continuous way. You just need to connect a source of your reviews to the tool, et voila—all your reviews get automatically added and categorized into topics. It makes feedback management more effective, so you can use your spare time to improve the customer experience.
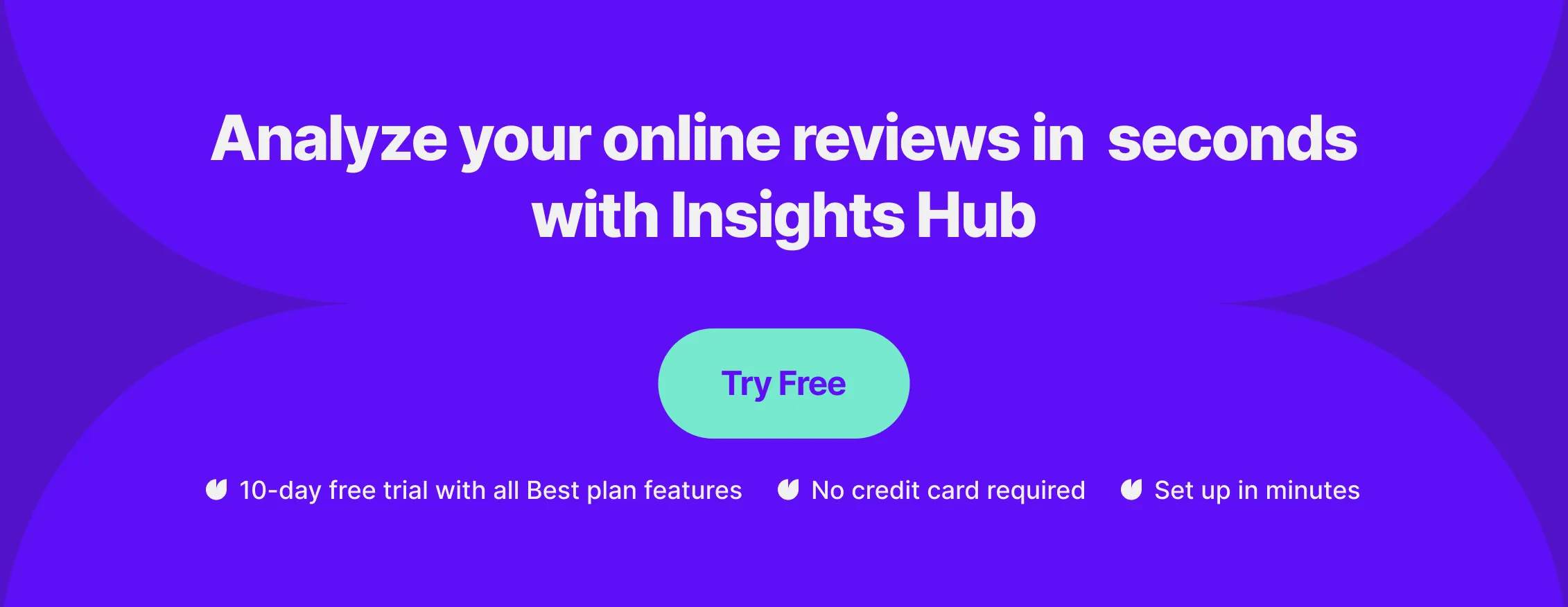
Customer interviews
Personal customer research interviews provide in-depth feedback. This method offers rich qualitative data, enabling you to explore customer behavior, experiences, and emotions in greater detail.
💡Tip: You can also use Insights Hub for transcribing your calls and interviews. It will automatically categorize them into topics as well. And if you have more questions—use Research Assistant, an AI chat that will use the aggregated feedback (only!) to find the answers.
Product analytics
Product analysis provides invaluable data on customer behavior insights. Tracking app usage, engagement, or how consumers interact with your product can add to a more comprehensive overview.
💡Tip: You can connect Survicate with many product analytics tools, such as Smartlook, Mixpanel, UXCam, or Amplitude, to create a synergy between the quantitative and qualitative data you collect.

Transaction data mining
Lastly, transaction data mining involves examining habitual buying behavior to detect patterns and trends. This quantitative method helps you understand customer buying habits and preferences, especially how often they purchase and how much they spend.
💡Tip: To get more qualitative information on the purchasing experience, you can consider adding a post-purchase template, so your customers can share in-depth feedback. ⤵️
Loyalty and retention measurement
Measuring customer loyalty and retention is essential for understanding the dynamic of your customer base and the health of your business relationships.
You can track several key metrics to analyze customer loyalty effectively:
1. Customer Satisfaction (CSAT)
Immediately after interactions use a CSAT survey and ask customers to rate their satisfaction on a scale from 1 to 5. The CSAT score is calculated as an average:

2. Net Promoter Score (NPS)
NPS survey categorizes customers into promoters, passives, and detractors based on their likelihood to recommend your services. This is often measured by one simple question:

3. Customer Retention Rate (CRR)
To calculate CRR, you need the number of customers at the start and end of a period and the number of new customers acquired during that period:

4. Repeat Purchase Ratio (RPR)
The RPR indicates the number of customers who have made more than one purchase over a given time frame.
- Existing repeat customers - Number of customers who have purchased before
- Total Customers - Overall number of customers in the same period
By analyzing these metrics, you develop a clearer picture of customer behaviors, their loyalty, and the likelihood of them remaining as returning customers.
Such data is vital for shaping retention strategies that cater to your customer's requirements and enhancing their overall experience with your brand.
Sentiment analysis
Sentiment analysis is a powerful tool that allows you to gauge public perception of your brand, product, or service.

This technique involves examining text data—be it reviews, social media conversations, or survey responses—to identify and categorize opinions expressed about your company. Here's how you can use sentiment analysis:
- Aspect-based analysis: Instead of evaluating whole responses, you can focus on specific 'aspects' to better understand customer feelings.
- Emotion tracking: By analyzing language, you can discern underlying emotions, whether positive, neutral, or negative, aiding you in understanding customer attitudes.
- Real-time feedback: Tools like Survicate offer immediate insight into customer reactions during product launches or marketing campaigns.
Benefits of sentiment analysis
- Improved customer understanding: Pinpoint areas of satisfaction or concern.
- Product development: Adjust or develop products based on direct customer feedback.
- Customer service: Tailor your approach to address common sentiments.
- Strategic decisions: Influence company direction using customer-driven data.
Tools and techniques:
- AI and machine learning: Use AI-powered tools such as Survicate’s Insights Hub to quickly analyze large volumes of data.
- Data fine-combing: Utilize in-depth analysis to tease out specific customer sentiments.
By integrating sentiment analysis into your strategy, you elevate your ability to respond to customer needs and shape experiences that resonate positively.
Remember, the goal is to understand and serve your customers better, and sentiment analysis brings you that much closer to achieving this.
Qualitative analysis
Qualitative analysis of customer behavior offers deep insights into your customers' motivations, perceptions, and experiences beyond mere statistics.
Customer journey mapping
With customer journey mapping, you can visualize the path your customers take from first awareness to final purchase and beyond. Each touchpoint allows qualitative data collection, revealing the emotions and thoughts that influence customer decision-making.
- Awareness: Understand what triggers your customer's search for a solution.
- Consideration: Examine the factors your customers evaluate when comparing options.
- Decision: Identify the pivotal reasons behind choosing your product or service.
- Customer retention: Analyze feedback for insights on customer loyalty and repeat purchase behavior.
Persona development
Developing detailed customer personas entails combining demographic, psychographic, and behavioral characteristics to represent your target audience segments.
Examples:
- Young Professional Pete might value time-saving features and quick customer service due to his busy lifestyle.
- Budget-conscious Brenda could prioritize cost-effectiveness and long-term value in her purchasing decisions.
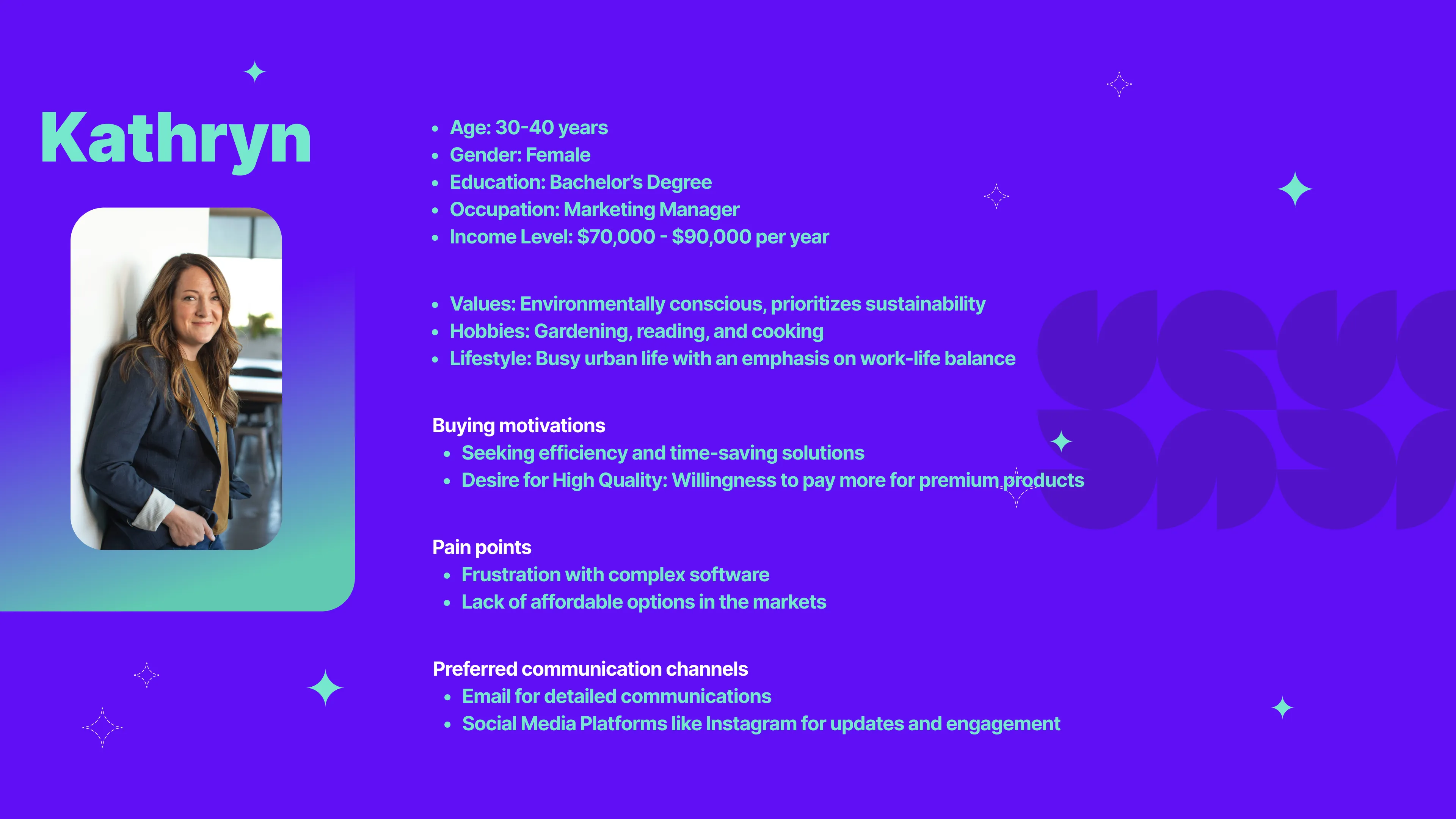
Crafting personas allows you to design strategies and communications to resonate with each segment's specific needs and preferences.
Quantitative analysis
Quantitative analysis in customer behavior uses numerical data to understand patterns, predict trends, and make data-driven decisions.
It employs statistical and machine learning algorithms to process large datasets and reveal insights into customer actions and preferences.
Statistical modeling
Statistical modeling is your foundation for interpreting customer behavior data. Specifically, regression analysis can help you determine the relationship between a customer’s purchasing frequency and variables such as price or marketing exposure.
For instance, a logistic regression might be used to predict the likelihood of a customer making a purchase based on their demographic data.
- Correlation analysis: Pinpoints the relationship between different customer actions, such as browsing and spending patterns.
- Time series analysis: This helps you track changes in customer behavior over time, which can be essential for seasonal trend analysis.
Machine learning applications
Machine learning takes your quantitative analysis to the next level by handling complex datasets that traditional statistical methods may struggle to process.
For customer behavior analysis, classification algorithms can categorize customers into different groups based on purchasing behavior, while clustering algorithms like K-means can segment customers by similarities in their shopping patterns without predefined categories.
- Predictive analysis: Utilizes algorithms like Random Forest or Gradient Boosting to forecast future behavior based on historical data.
- Pattern recognition: Identifies recurring sequences in customer behavior, enabling personalized marketing efforts.
Example: Using a machine learning model, you can predict an individual's Customer Lifetime Value (CLV) by analyzing their historical transaction data and engagement metrics.
Behavioral segmentation
When tailoring your marketing efforts, understanding your customers' behaviors is crucial. Behavioral segmentation is a powerful analytical approach that groups your customers based on their actions, such as the products they choose, the frequency of their purchases, and their loyalty to your brand.
Key aspects of behavioral segmentation:
- Purchase behavior: Observe how customers make buying decisions. Consider the types of products they prefer and the motivational factors behind their purchases.
- Usage rate: Identify customers based on how often they use your product. Categories might include 'heavy,' 'medium,' or 'light' users.
- Benefit sought: Tailor your offerings by understanding the primary benefit each customer seeks from your product, whether it's convenience, durability, or cost-effectiveness.
- Customer loyalty: Assess the loyalty levels of customers. Loyal customers can provide insights into your product's strengths and help you build long-term relationships.
Employing predictive modeling can enhance behavioral segmentation by using data about past behaviors to forecast future patterns.
Psychographic segmentation
Psychographic segmentation divides your market into groups based on psychological characteristics—personality traits, values, interests, lifestyles, and attitudes.
This approach complements demographic segmentation, focusing more on the "why" behind consumer behaviors, whereas demographics address "who" your customers are.
Key variables in psychographic segmentation:
- Personality: Involves categorizing consumers based on traits like extroversion, agreeableness, or neuroticism.
- Values: Centers on what your audience holds important, such as sustainability or family.
- Lifestyle: Looks at day-to-day life aspects, hobbies, and activities.
- Interests: Targets what your customer is passionate about, ranging from tech to fashion.
- Social class: Addresses the economic or social hierarchies impacting consumer choices.
Understanding these psychographic factors allows you to tailor your marketing strategies to resonate more deeply with specific customer segments. For example:
- Personality could be leveraged to customize messaging that appeals to an adventurous spirit.
- Marketing campaigns emphasizing values might focus on eco-friendly products for environmentally conscious groups.
To implement effective psychographic segmentation:
- Identify psychographic variables relevant to your product or service.
- Use surveys, interviews, and data analytics to gather psychographic data.
- Analyze the data to form distinct segments.
Realize that customer preferences are not static—they evolve. Regularly update your psychographic segments to reflect these changes and maintain their relevance.
How to run customer behavior analytics and data interpretation?
Customer behavior analysis relies on insightful data interpretation. It allows you to understand past trends, predict future behavior, and make informed decisions.
Descriptive analytics
Descriptive analytics provides a clear view of your customers' past actions. This involves collecting data from various sources, such as sales records, website interactions, and customer feedback, to paint a picture of historical behavior.
Key elements include:
- Patterns of customer buying behavior: Frequency and types of products bought.
- Customer engagement metrics: Website visits, content views, and social media interaction.
Predictive analytics
Predictive analytics uses historical data to predict customer behaviors and trends. Machine learning algorithms and statistical models are typically employed for this analysis.
Important predictive measures:
- Future consumer buying behavior: Likely products customers will buy.
- Customer churn probability: Likelihood of customers discontinuing service.
Prescriptive analytics
Prescriptive analytics goes one step further by suggesting actions you could take to influence desired customer behavior. It uses actionable insights from descriptive and predictive analytics to recommend strategic decisions.
Prescriptive tactics encompass:
- Product recommendations: Based onthe customer's buying history.
Run a customer behavior analysis with Survicate
Understanding customer behavior is essential for any business looking to improve its customer experience and drive growth.
By analyzing customers' interactions with your business, from purchasing patterns to feedback, you gain valuable insights that can guide your marketing strategies, product development, and overall customer engagement.
Enter Survicate. With features like AI-driven surveys, feedback categorization, and native integrations, Survicate makes the process of collecting and interpreting customer data effortless.
With insights gained from Survicate, you can craft more personalized experiences, predict future trends, and make well-informed decisions that resonate with your customers.
Take the first step towards unlocking the full potential of your customer data. Try Survicate for free with a 10-day trial that offers all the Best Plan features, and experience firsthand how it can transform your approach to customer behavior analysis.
Frequently Asked Questions
In this section, you'll find concise answers to common inquiries about customer behavior analytics, which can empower your marketing efforts, inform product development, and sharpen your sales predictions.
How can customer behavior analytics improve marketing strategies?
By analyzing customer behavior, you can tailor your marketing strategies to meet different customer segments' specific needs and preferences.
This targeted approach can lead to higher engagement and conversion rates.
What are the key tools used for consumer behavior analysis?
Key tools for analyzing customer behavior include data management platforms (DMPs), customer relationship management (CRM) systems, web analytics tools, and specialized software that uses machine learning algorithms to identify patterns in customer data.
In what ways can analyzing customer behavior benefit a business?
Analyzing consumer behavior benefits your business by revealing insights into customer preferences, predicting future buying trends, and allowing for personalization of marketing efforts, which can increase customer loyalty and revenue.
What are the primary types of customer behavior models used in sales predictions?
The primary customer behavior models used in sales predictions are the AIDA model (Awareness, Interest, Desire, Action), the buyer's journey (Awareness, Consideration, Decision), and RFM (Recency, Frequency, Monetary) analysis.
How is Python utilized in the analysis of customer behavior data?
Python is utilized to analyze customer behavior data through its various data analysis libraries like Pandas and NumPy and machine learning libraries such as Scikit-learn, which enable the processing, analysis, and predictive modeling of large datasets.
Can you provide an example of how customer behavior analysis impacts product development?
An example of customer behavior analysis impacting product development is when data reveals a significant interest in a feature that competitors lack.
This insight can drive the creation of new product offerings or enhancements to existing ones to meet an unaddressed need in the market.